In the world of academia, dissertations and capstones serve as the pinnacle of a student’s journey. These culminating projects not only showcase a student’s mastery of a particular subject but also aim to contribute novel insights to the wider scholarly community. However, within the pages of these extensive research documents lies a treasure trove of data waiting to be unlocked. Dissertation data analysis holds the key to extracting meaningful conclusions, identifying trends, making evidence-based recommendations, and ultimately pushing the boundaries of knowledge.
The process of dissertation data analysis involves examining and interpreting the vast amounts of information collected during the research phase. It entails meticulous organization, systematic examination, and data-driven reasoning to unveil the hidden gems within the dataset. By engaging in this analytical journey, researchers can uncover patterns, relationships, and correlations that might have otherwise gone unnoticed. Moreover, dissertation data analysis allows for the validation or refutation of hypotheses put forth, enabling the researcher to draw robust conclusions based on empirical evidence.
Statistics Help For Dissertation
Undoubtedly, the importance of dissertation data analysis can hardly be overstated. It empowers researchers to move beyond mere observations to construct an intricate narrative backed by data. Furthermore, it provides an opportunity to delve into the complexities of the research questions at hand, exploring various dimensions to shed light on the underlying phenomena. By meticulously analyzing and synthesizing the gathered data, researchers can generate new insights, challenge existing theories, and contribute to the overall body of knowledge within their field of study.
In the pages that follow, we will embark on a journey of cracking the code of dissertation data analysis. We will explore the techniques, methodologies, and tools employed to extract meaningful insights from the wealth of information available. Through this exploration, we aim to equip both seasoned researchers and aspiring scholars with the necessary skills and knowledge to navigate the often-daunting realm of dissertation data analysis. So, let us unlock the potential lying dormant within these dissertations and capstones and unearth the valuable insights waiting to be discovered.
Understanding Dissertation Data Analysis
Dissertation data analysis plays a pivotal role in uncovering valuable insights within dissertations and capstone projects. It involves the systematic examination and interpretation of data collected during the research process, aiming to derive meaningful conclusions and support or refute research hypotheses. Through data analysis, researchers can transform raw data into valuable knowledge, shedding light on trends, patterns, and relationships that might not be immediately apparent. Let’s delve deeper into the intricacies of this crucial stage of the research process.
Firstly, dissertation data analysis involves the meticulous organization and preparation of data for analysis. Researchers need to clean and validate the data, ensuring its accuracy and integrity. By removing inconsistencies and errors, researchers can enhance the reliability of the analysis and its outcomes. This step is crucial in providing a solid foundation for subsequent analysis techniques and ensuring the validity and credibility of the research findings.
Next, researchers employ various statistical techniques during the analysis phase, allowing them to explore, summarize, and interpret the data effectively. These techniques can range from simple descriptive statistics, such as calculating means and frequencies, to more complex inferential statistics, such as hypothesis testing and regression analysis. The aim is to identify significant patterns, trends, and relationships within the data, providing valuable insights into the research topic. By applying appropriate statistical methods, researchers can draw reliable conclusions and make informed decisions based on the data they have collected.
Moreover, data analysis in dissertations often involves the use of specialized software tools and programming languages. These tools facilitate the exploration and manipulation of data, enabling researchers to conduct advanced analyses and generate visual representations of the findings. Statistical software packages, such as SPSS, R, or SAS, are commonly utilized, providing a comprehensive set of tools to analyze and interpret data effectively. By harnessing the power of these technologies, researchers can navigate through large datasets, uncover hidden relationships, and present their findings in a clear and concise manner.
In conclusion, dissertation data analysis is an indispensable step in the research process. It involves the systematic examination and interpretation of data, enabling researchers to uncover valuable insights within their dissertations and capstone projects. By employing various statistical techniques, ensuring data accuracy, and utilizing specialized software tools, researchers can extract meaningful conclusions from their data, contributing to the advancement of knowledge within their respective fields.
Effective Strategies for Data Interpretation
In order to effectively interpret dissertation data analysis, there are several strategies that can be employed. These strategies can help researchers extract valuable insights from their data and make informed conclusions. Here are three key approaches that can enhance the interpretation process:
Visualization Techniques: Visualizing data is a powerful way to make sense of complex information. Using charts, graphs, and diagrams can help researchers identify patterns, trends, and relationships within their data. By presenting data visually, researchers can easily communicate their findings to others and gain a deeper understanding of the underlying patterns in the data.
Comparative Analysis: Engaging in comparative analysis can provide valuable insights into the data. This involves comparing different variables, groups, or time periods to identify similarities, differences, and correlations. By analyzing data from multiple perspectives, researchers can gain a holistic view of the phenomenon under investigation and uncover hidden connections that may not be immediately evident.
Contextualization: Understanding the context in which the data was collected is essential for accurate interpretation. Researchers should consider the specific research questions, objectives, and theoretical frameworks that guided the study. Additionally, examining relevant literature and previous studies can provide a broader context for interpreting the data, helping to validate and refine the findings.
By employing these effective strategies for data interpretation, researchers can unlock valuable insights from their dissertation data analysis. Visualizing the data, conducting comparative analysis, and contextualizing the findings are essential components of the interpretation process, allowing researchers to draw meaningful conclusions and contribute to the existing body of knowledge.
Key Considerations for Conducting Dissertation Data Analysis
When it comes to conducting dissertation data analysis, there are several key considerations to keep in mind. These considerations can greatly impact the quality and validity of your findings. By taking these factors into account, you can ensure a thorough and reliable analysis of your dissertations and capstones.
Data Collection and Organization: Before diving into the analysis phase, it is crucial to have a clear plan for data collection and organization. This involves identifying the relevant variables and ensuring they are properly measured and recorded. Designing a structured framework for data entry and storage will enhance the efficiency and accuracy of your analysis.
Research Questions and Hypotheses: Defining clear research questions and hypotheses is essential for guiding your data analysis process. These questions should be aligned with the objectives of your dissertation or capstone, and they should drive the analysis towards specific insights. By clearly articulating your research questions and hypotheses, you can focus your efforts on extracting meaningful findings from your data.
Appropriate Statistical Techniques: Choosing the right statistical techniques for your data analysis is crucial to ensure valid and reliable results. Depending on the nature of your data and research questions, you may need to employ various statistical methods such as regression analysis, chi-square tests, or factor analysis. By selecting the appropriate techniques and applying them correctly, you can uncover valuable insights and draw meaningful conclusions from your dissertation data.
In conclusion, conducting effective dissertation data analysis requires careful consideration of various factors. From data collection and organization to defining research questions and utilizing suitable statistical techniques, each step plays a critical role in unlocking valuable insights from dissertations and capstones. By following these key considerations, researchers can enhance the rigor and credibility of their analyses, contributing to the advancement of knowledge in their respective fields.
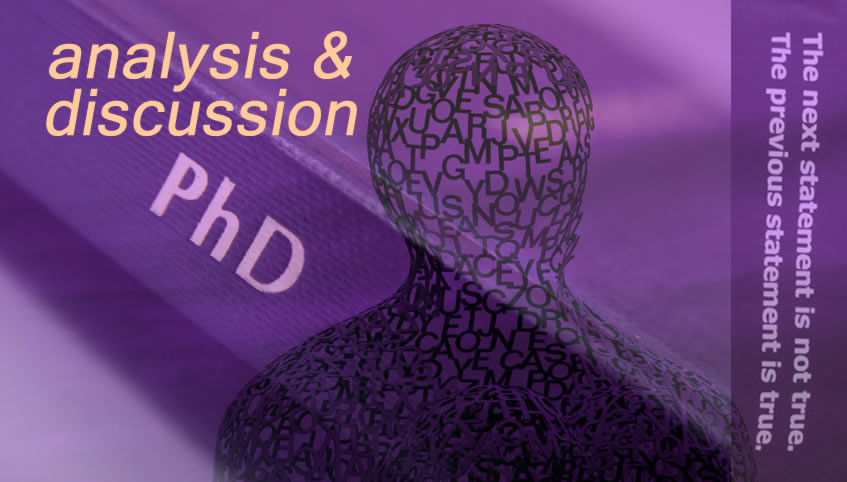